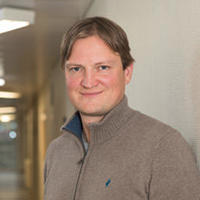
Prof. Alexandre Tkatchenko
Head of the Research Group
Alexandre Tkatchenko is a Professor of Physics and Chair of Theoretical Chemical Physics at Uni.lu. Tkatchenko also holds a distinguished visiting professor position at the Technical University of Berlin and has co-founded two deeptech startups. He has delivered more than 450 invited talks, seminars and colloquia worldwide and served on the editorial boards of four society journals: Science Advances (AAAS), Physical Review Letters (APS), Chemical Science (RSC), and the Journal of Physical Chemistry Letters (ACS). Tkatchenko has received a number of awards, including APS Fellow from the American Physical Society, Fellow of the Royal Society of Chemistry (FRSC), Gerhard Ertl Young Investigator Award of the German Physical Society, Dirac Medal from the World Association of Theoretical and Computational Chemists (WATOC), Feynman prize from the Foresight Institute, van der Waals prize of the international conference on non-covalent interactions (ICNI), and five flagship grants from the European Research Council: a Starting Grant in 2011, a Consolidator Grant in 2017, an Advanced Grant in 2022, and Proof-of-Concept Grants in 2020 and 2023. During free time, he tinkers with both gravity and quantum mechanics.
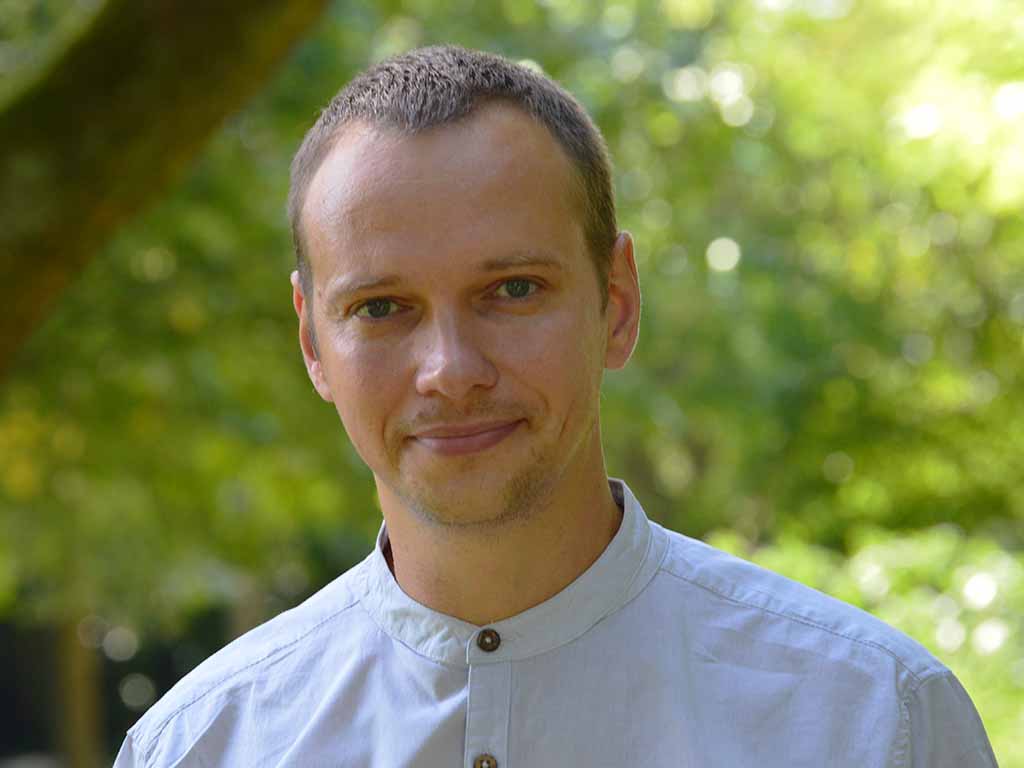
Igor Poltavskyi
Research Scientist; Team Leader on Machine Learning
Research Interests: Statistical physics, imaginary-time path integral methods, nuclear quantum effects, ab initio simulations
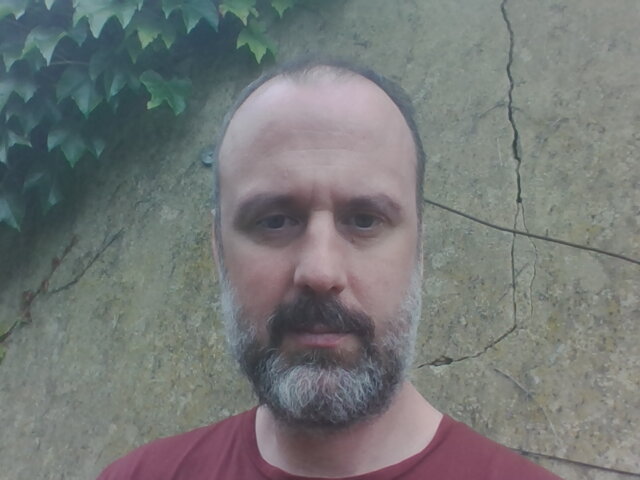
Josh Berryman
Research Scientist; Team Leader on Biomolecular Modeling
Josh Berryman has lifelong commitments to machine learning and to interdisciplinary physics, his highlight achievements include pioneering the use of neural networks for Go in 2000, developing multimedia-capable wireless devices at Imagination Technologies, doing a PhD at Leeds University in biomolecular modelling in 2010, and proposing and verifying a mechanism for COVID neurotoxicity in 2022. He is a member of the college of fellows of Luxembourg's Institute for Advanced Studies, and has won and managed multiple Luxembourgish research grants including CORE and CORE Junior, as well as EU-JPND funding for investigating the overlap between COVID and neurodegenerative disease [covaminf.org].
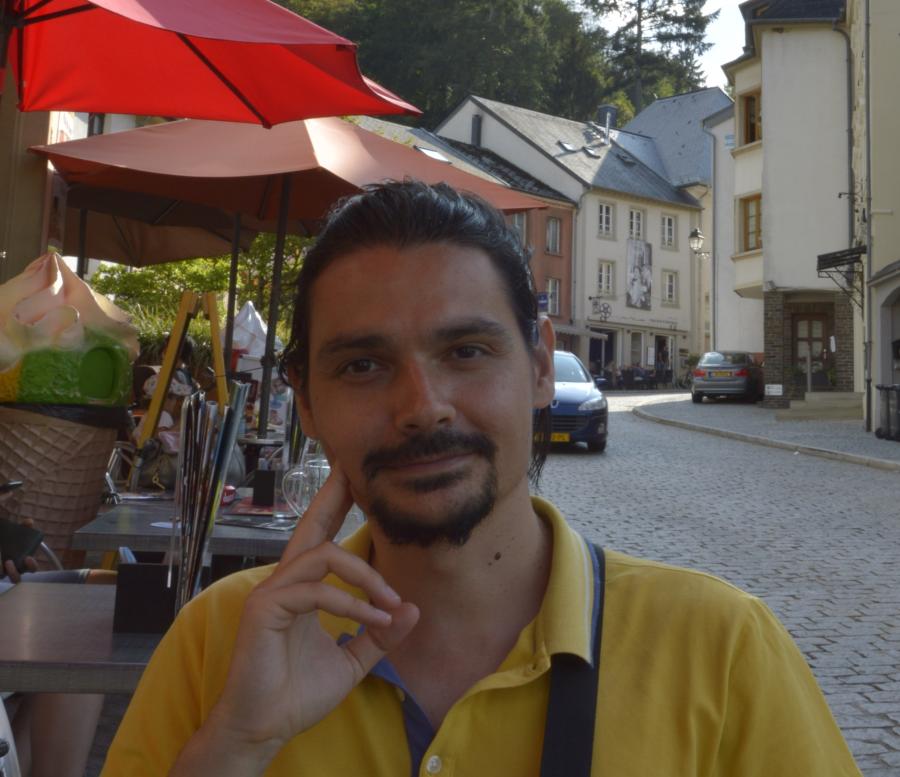
Matteo Barborini
Research Scientist; Team Leader on HPC and Computational Quantum Mechanics
Researcher in Computational Chemical Physics working at the HPC Platform of the University of Luxembourg with many years of experience in the development and implementation of quantum Monte Carlo methods, and in the application of several Computational Methods in Quantum Chemistry and Solid State Physics. During his career he contributed to the development of Prof. Sandro Sorella's code TurboRVB and since 2017 he has been the initiator and main developer of the Quantum MeCha (QMeCha) quantum Monte Carlo package. His main interest is in the development of explicitly correlated wave functions for the solution of the many-body time independent Schrodinger equation for molecular systems. Recently, he has contributed to the supervision of PhD students in projects related to Molecules-Positron meta-stable states and Molecules-QDOs embedding procedures.
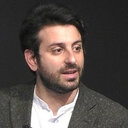
Matteo Gori
Research Scientist; Team Leader for Statistical Mechanics
Matteo Gori is a theoretical physicist exploring the interplay between statistical mechanics, quantum-classical mixed dynamics, and many-body van der Waals interactions in complex systems. His research focuses on uncovering how electrodynamic interactions—both classical and quantum—govern the dynamical organization of biomolecular systems. By combining differential geometry, quantum field theory, and advanced statistical methods with numerical simulations, he investigates emergent collective phenomena, bridging fundamental physics with real-world biological complexity.
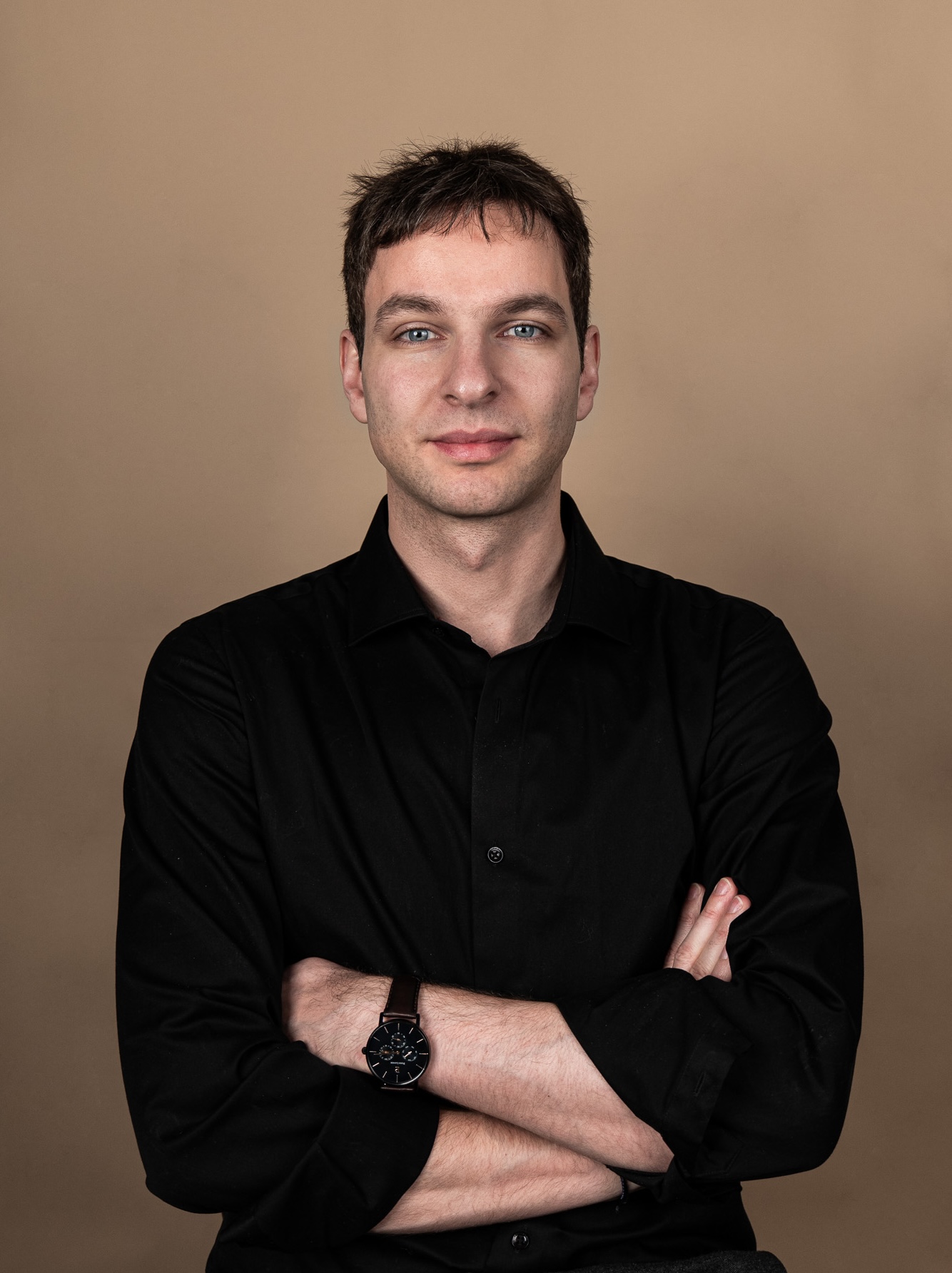
Matthieu Sarkis
Research Scientist; Team Leader for Theoretical Quantum Mechanics
His research focuses on multipartite entanglement in quantum information theory and the application of quantum machine learning to quantum chemistry, with a particular emphasis on long-range interactions. His work explores fundamental questions at the intersection of quantum many-body physics and quantum information. He is also interested in QFT and in particular Conformal Field Theories in 2 dimensions.
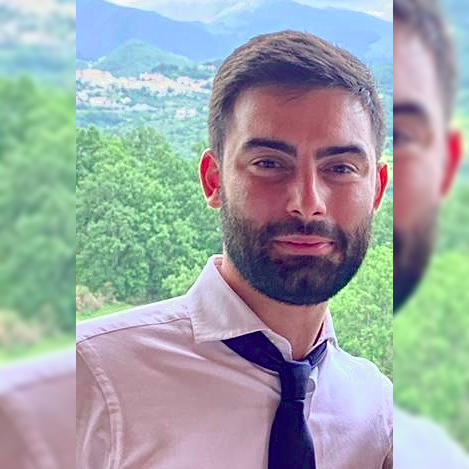
Loris Di Cairano
Post-Doc
I explore the intricate boundary between equilibrium and criticality, where phase transitions and collective phenomena emerge across scales--from high-energy physics to macroscopic systems. My work merges rigorous mathematical frameworks with fine-grained numerical simulations, advancing the microcanonical ensemble to probe the deep mechanisms of phase transitions. By leveraging recent and unexpected developments in statistical mechanics, I develop novel numerical procedures and theoretical frameworks based on differential geometry to characterize phase transitions, thus blending analytical insights with computational lattice techniques. Beyond classification, my goal is to unveil transitions and cooperative behaviors emerging in physical systems.
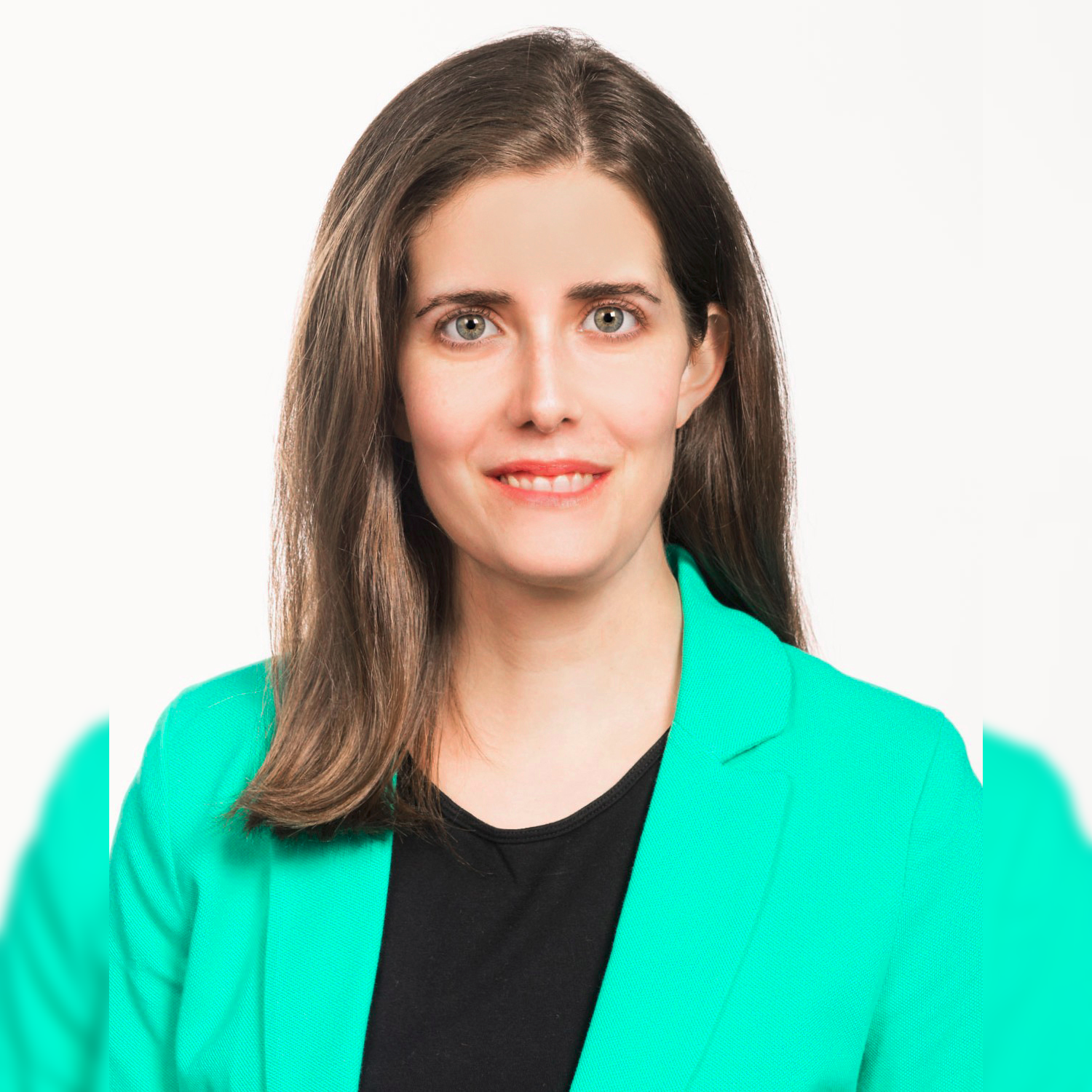
Ariadni Boziki
Post-Doc
Ariadni Boziki is a Chemical Engineer who earned her diploma in Chemical Engineering from the National Technical University of Athens in 2014. That same year, she joined École Polytechnique Fédérale de Lausanne (EPFL) in Switzerland, where she completed her Ph.D. in computational chemistry and materials science in 2019. Following her Ph.D., she continued as a postdoctoral researcher at EPFL and later worked as a scientific research associate at the Swiss Institute of Bioinformatics. She then joined the research group of Prof. Alexander Tkatchenko at the University of Luxembourg. Her research integrates a diverse range of computational chemistry techniques, including ab initio and classical molecular dynamics simulations, Monte Carlo simulations, and ground- and excited-state calculations using methods such as DFT and wavefunction-based approaches. Through these methodologies, she investigates the properties of materials. She has worked extensively on halide perovskites, polymer/solid interfaces, and biomolecules such as proteins. In Tkatchenko's group, her research focuses on molecular crystals; materials of significant interest to the pharmaceutical industry due to their use in drug formulations. As part of this work, she developed THeSeuSS, an automated platform for simulating vibrational spectra of molecules and solids. To further enhance and accelerate vibrational spectrum simulations, she is also integrating machine learning techniques into her research.
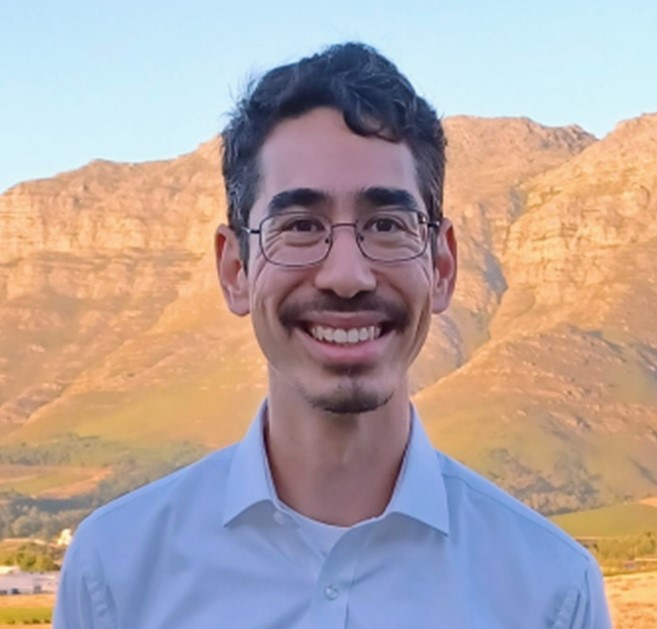
Dahvyd Wing
Post-Doc
Current interests: Development of interatomic potentials for large systems using neural networks combined with simple models of long-range physics, such as the many-body dispersion (MBD) method
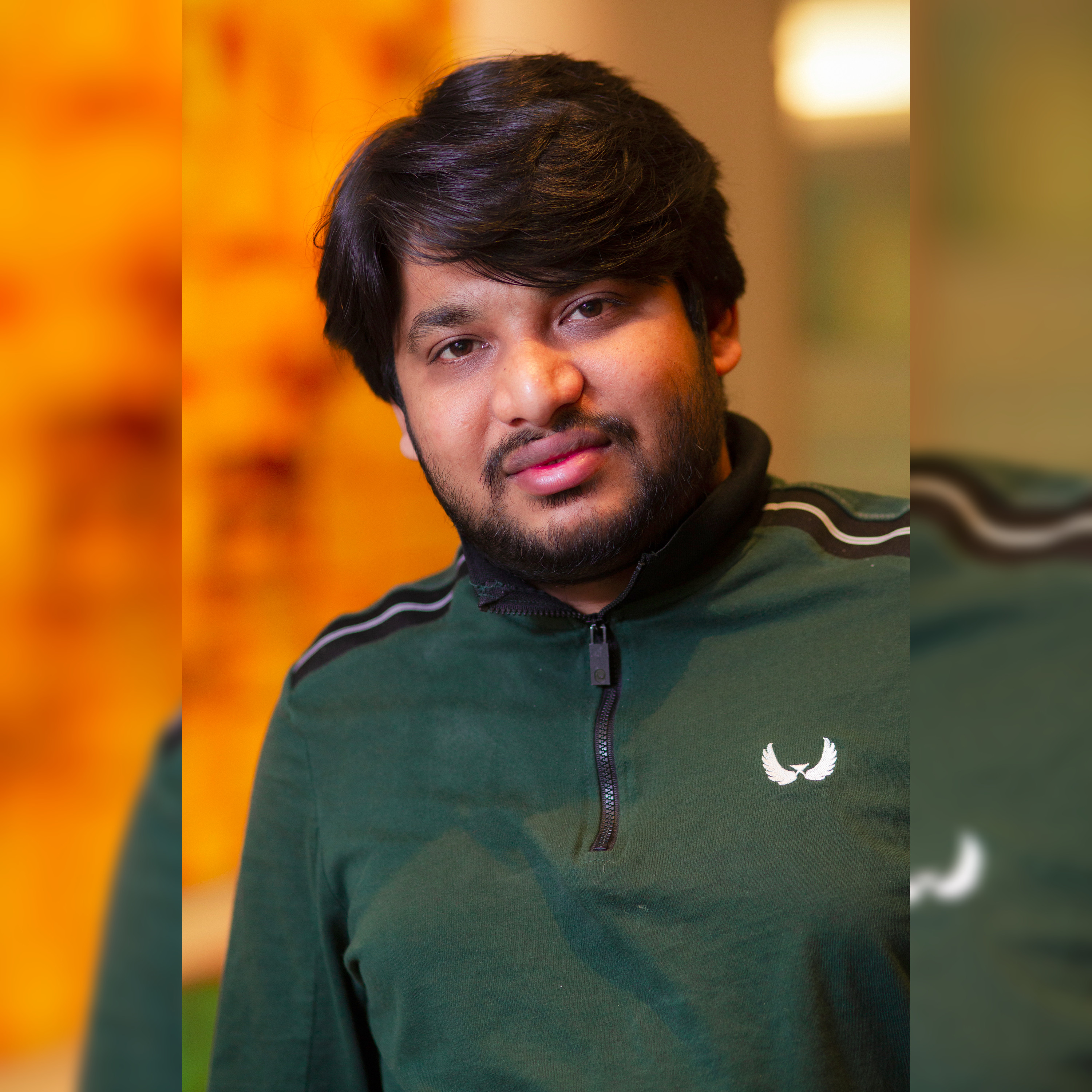
Apurba Nandi
Post-Doc
Expertise: Theoretical Chemistry, Reaction Dynamics, Machine-Learning, Electronic Structure Theory, Molecular Dynamics, Nuclear Quantum Effects
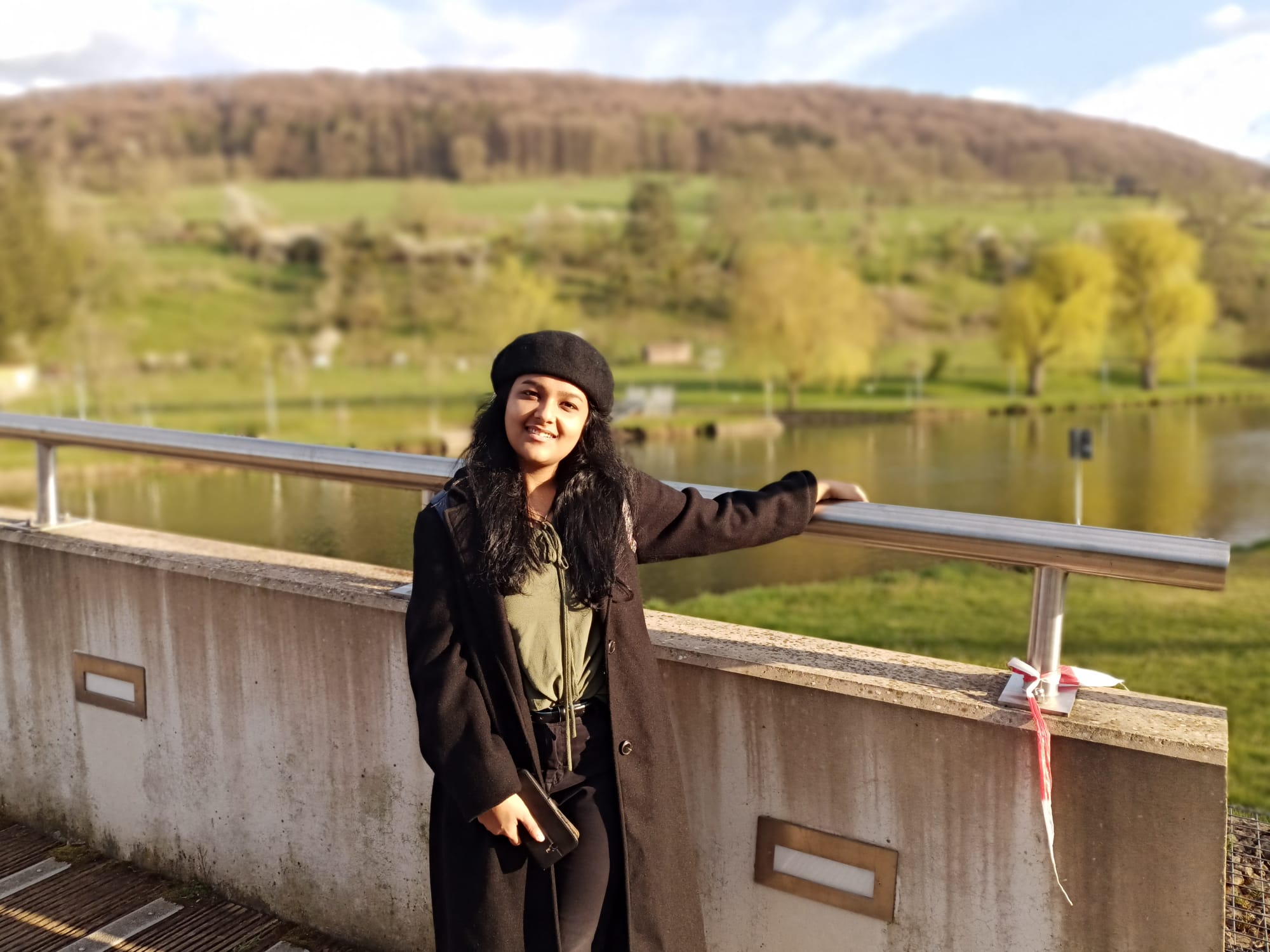
Ashmita Bose
Post-Doc
I have a background in Physics, holding both a Bachelor's and Master's degree, with a Ph.D. focused on unconventional computing using nonlinear chemical reactions. My current research lies at the intersection of machine learning and quantum chemistry for in-silico drug discovery. I apply advanced computational techniques, including Density Functional Theory (DFT) and Density Functional Tight Binding (DFTB), to analyze the quantum properties of drug-like molecules. By integrating these quantum features into machine learning models, I aim to improve the accuracy and reliability of predictive frameworks, contributing to more efficient drug design and discovery.
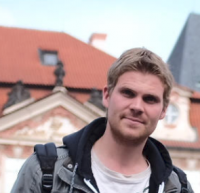
Daniel Bonhenry
Post-Doc
Expertise: Molecular dynamics simulations of biomolecules, Free-energy calculations, Molecular modelling (Homology modelling, docking, …)
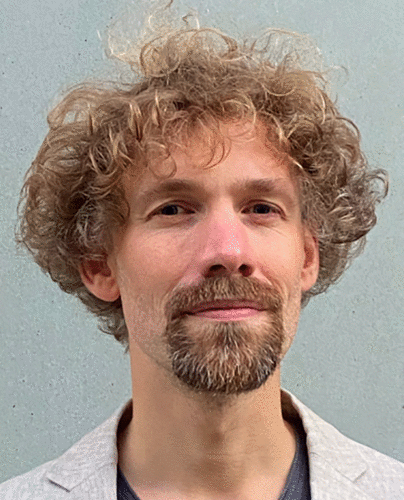
Florian Bruenig
Post-Doc
Florian Brünig is a theoretical physicist trained at Freie Universität Berlin with a strong focus on molecular biophysics. During his PhD he worked on simulations and stochastic modelling of proton dynamics and vibrational spectroscopy in water and biological environments as an integral part of the Collaborative Research Center 1078 "Protonation Dynamics in Protein Systems". His investigation of large protein complexes in experiments during the BSc, and the analysis of electron tomography data of single cells at Zuse Institute add to his broad background on biophysical research. His several publications showcase his expertise across various fundamental methods in statistical physics, i.e. linear response, projection and rare-event sampling, in combination with quantum chemical simulations techniques, as well as his effort in active interdisciplinary collaboration with experimental groups. The work has been honoured by several invited presentations, including at international conferences and universities, in addition to regular participation at numerous international conferences and workshops. His current interests extend to machine learning models for atomistic simulations of bio and soft matter systems and stochastic modeling of long-range interactions.
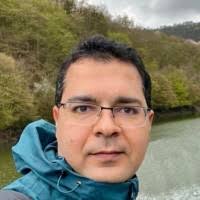
Reza Karimpour
Post-Doc
Have you ever wondered how invisible quantum forces shape the world around us? I do, every day! I'm a theoretical physicist exploring the forces and interactions that emerge from quantum mechanical fluctuations of electromagnetic and matter fields. My research, grounded in quantum electrodynamics and quantum field theory, covers a range of fascinating topics, from the Casimir effect in curved spaces and/or between macroscopic objects to Casimir-Polder and van der Waals forces in atomic, molecular, and low-dimensional material systems. I also investigate how external bodies and fields influence intermolecular interactions. While my work is rooted in theory, I like thinking about how these quantum effects could be observed in real-world experiments. What would it take to measure them? How could we design experiments to test these predictions? These questions drive my curiosity and motivate my research.
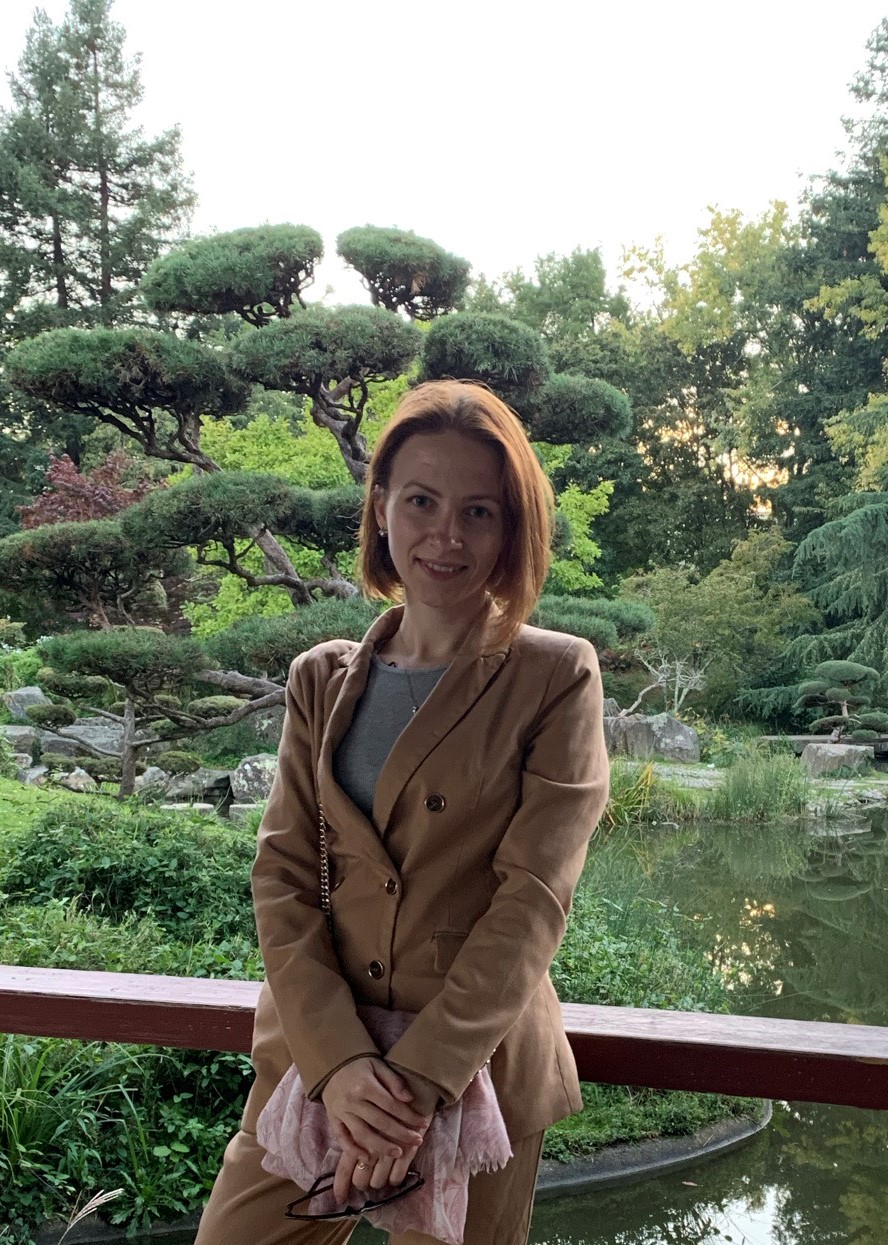
Iryna Knysh
Post-Doc
Iryna Knysh is a theoretical chemist with a background in organic chemistry and chemical nanoengineering. During her PhD, she explored the accuracy of the Bethe-Salpeter equation and many-body Green's function formalism (BSE/GW) in modeling the excited-state properties of organic dyes. Her expertise includes the theoretical prediction of excited-state properties and vibrationally resolved spectra using various QM methods, ranging from TD-DFT to coupled-cluster theories. She is currently involved in projects focused on modeling material and molecular properties using modern machine learning algorithms. Her research interests lie at the interface of machine learning and excited-state QM approaches, aiming to design more efficient optoelectronic and photovoltaic materials.
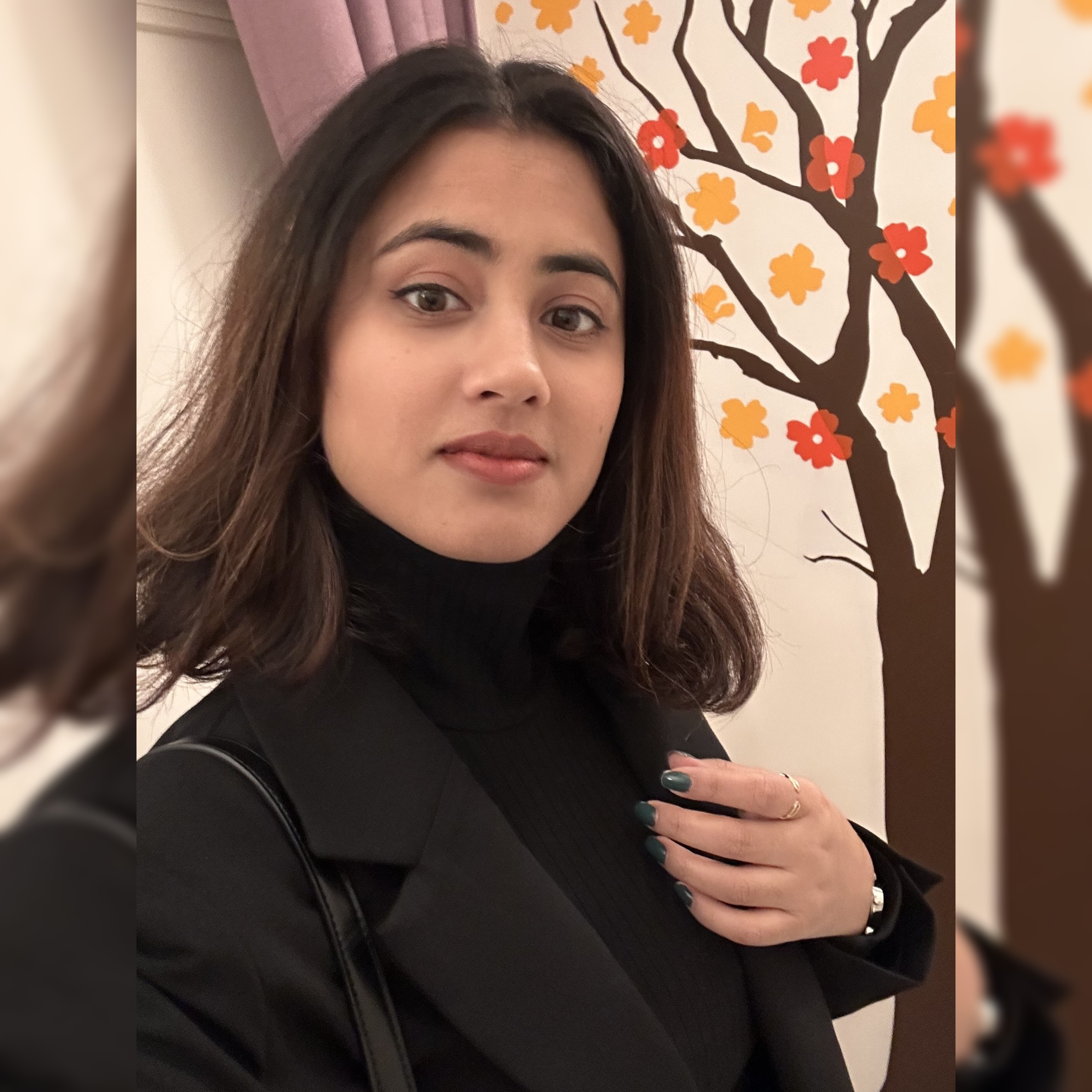
Naziha Tarannam
Post-Doc
I have obtained my PhD at the Ben-Gurion University of the Negev, Israel in 2022 under the supervision of Prof. Sebastian Kozuch. During my PhD, I focused on developing a quantum chemical model for assessing the kinetics of catalytic cycles and applying it to various organometallic and non- covalent organocatalytic processes. After submitting my PhD thesis, I worked with Prof. Dan T. Major at Bar-Ilan University, Israel, on a short-term postdoctoral position, where I investigated the hydrolysis of toxic nerve agents occurring at the active site of the enzyme Phosphotriesterase. In October, 2024, I joined the TCP group at the University of Luxembourg as a Postdoctoral researcher. My current research interests include developing and refining Machine Learning Force Fields (MLFFs) for accurate depiction of diverse biomolecular dynamics, in comparison to classical force fields. Additionally, I am interested in understanding the role of long-range van der Waals interactions in large biomolecules.
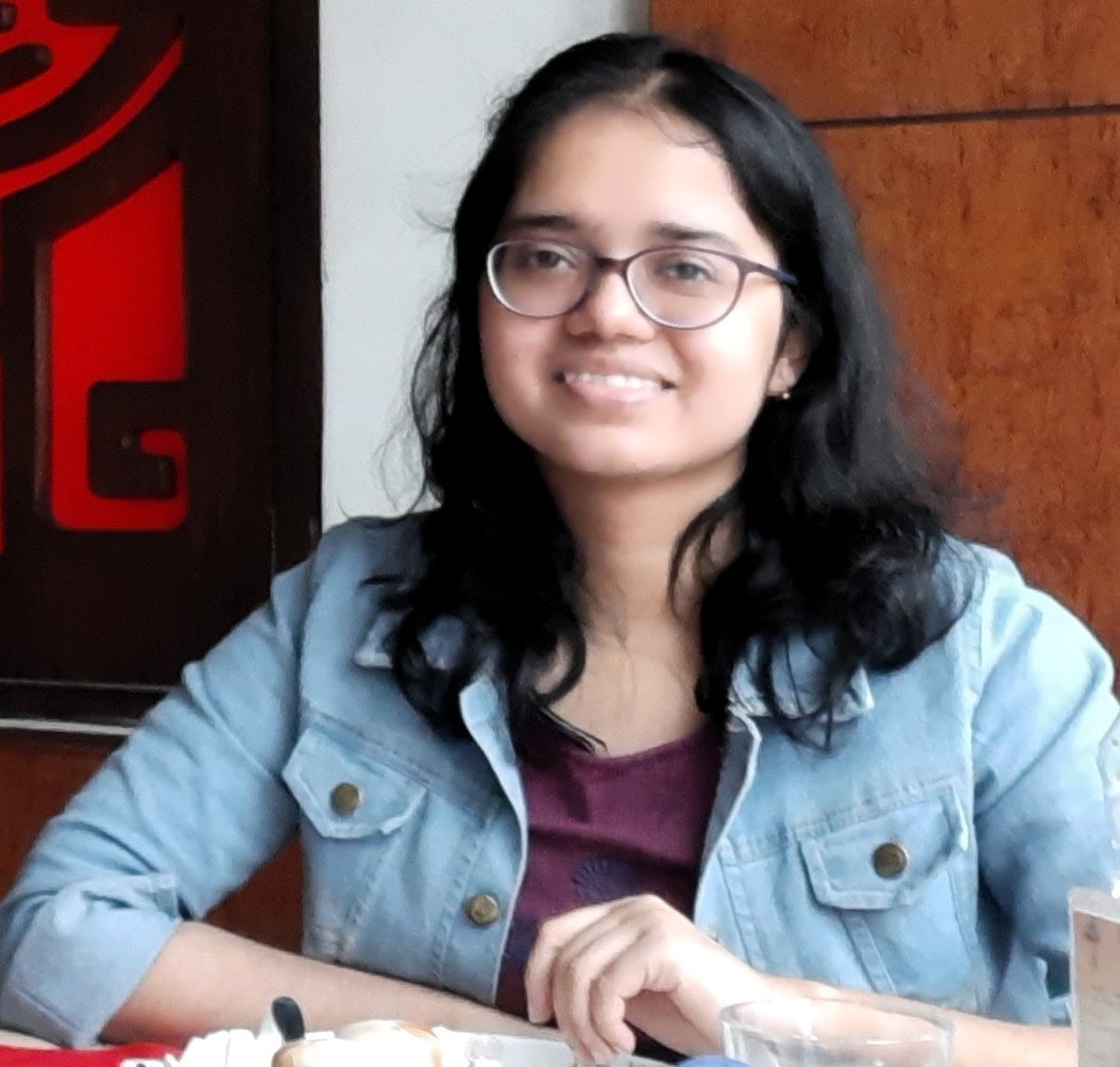
Upala Mukhopadhyay
Post-Doc
Upala Mukhopadhyay is a cosmologist exploring various inter-connected topics in cosmology, e.g., Dark Energy, Dark Matter, modified gravity, 21cm Hydrogen line in reionization epoch, Primordial Black Holes, Gravitational Waves etc. Her research mainly focuses on the theoretical and phenomenological study of the late time acceleration of the Universe, Dark Energy and cosmic tensions (namely, the Hubble tension, the S8 tension, the tension of high redshift massive galaxies reported by James Webb Space Telescope). Along with analytical calculations, she also analyses large cosmological data sets related to type-Ia supernovae, baryon acoustic oscillations, cosmic microwave background, growth of cosmic structures etc. Since cosmology have arrived in a data driven era, she is interested to explore machine learning techniques in cosmology to better understand the unknown components of our Universe by unifying experimental results and theoretical aspects.
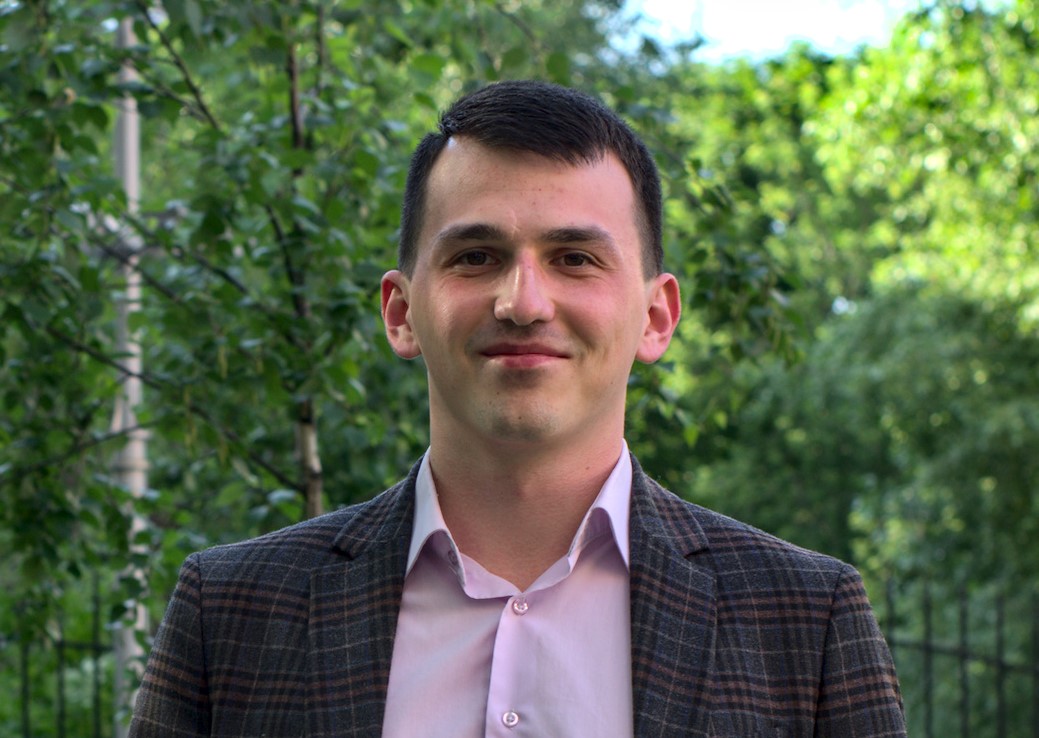
Almaz Khabibrakhmanov
Post-Doc
I graduated from the Moscow Institute of Physics and Technology (MIPT) in 2020, where I developed a strong background in chemical physics and computational materials science. In 2021, I joined the research group of Prof. Alexandre Tkatchenko at the University of Luxembourg, where I have recently completed my PhD. My doctoral research focused on designing simple yet accurate models for van der Waals (vdW) dispersion interactions using quantum Drude oscillators (QDOs). One of the key outcomes was the development of universal interatomic vdW-QDO potentials – efficient tools that have already found practical applications in machine-learning force fields (MLFFs), helping to model long-range interactions typically missing from neural network potentials. On a more fundamental level, my work revealed how vdW dispersion can polarize electron density – an effect often overlooked but critical for large, polarizable systems. These insights open exciting opportunities for developing next-generation "dispersion-consistent" density functionals. Currently, I am continuing this line of research as a short-term PostDoc in the group, with a focus on bridging the gap between accurate quantum mechanical models and practical applications in (bio)molecular simulations.
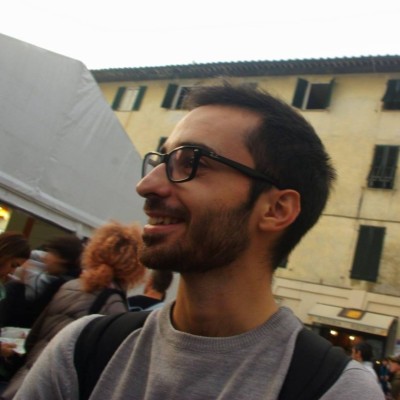
Alessio Fallani
PhD Student
Current Project: Design and validation of novel ML-based tools benchmarked on quantum mechanical reference data of chemical reactions, to be used to accurately predict components of the vast chemical reaction space emphasizing transition state features.
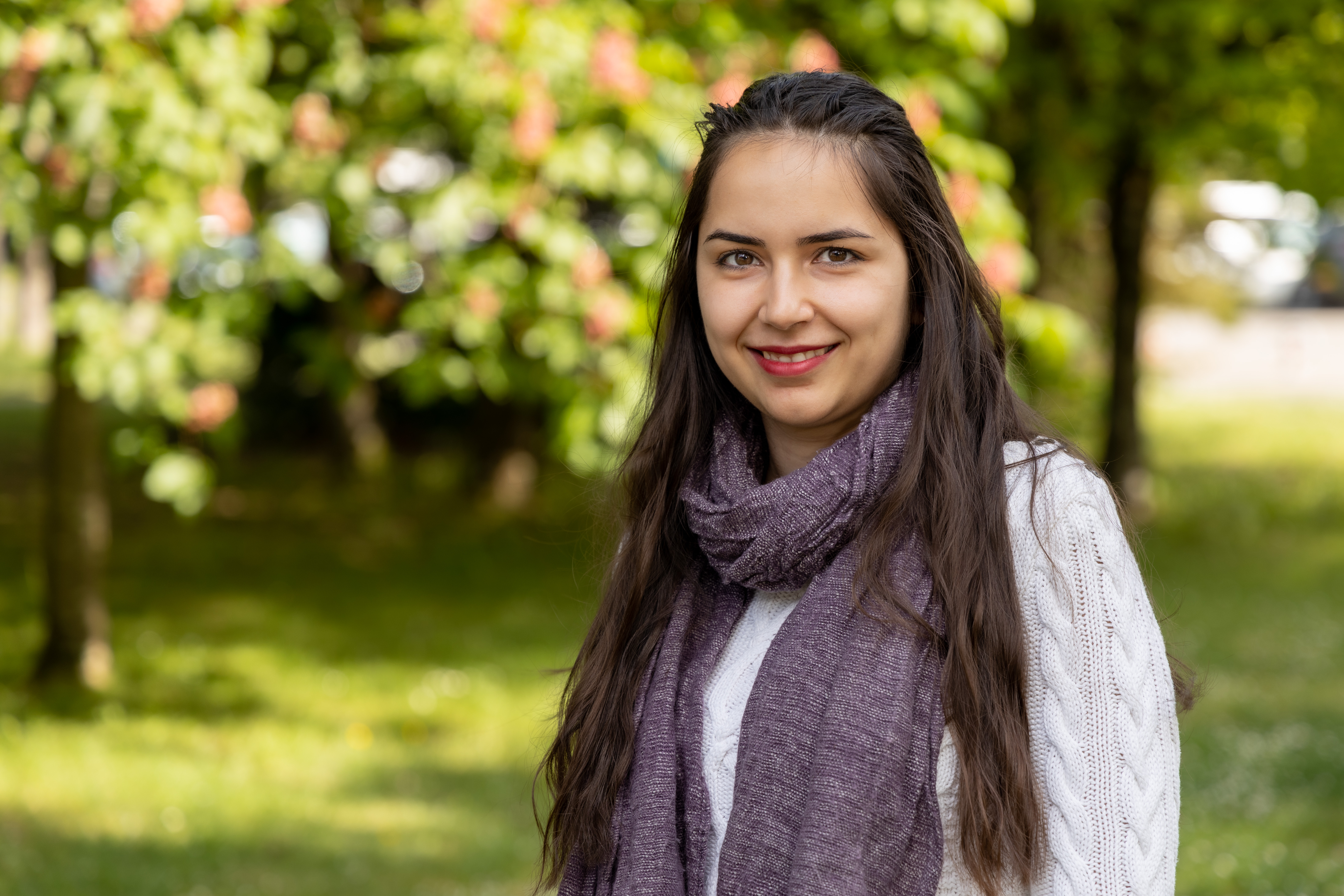
Mirela Puleva
PhD Student
The interdisciplinary PhD project "Approaching Quantum Mechanical Accuracy for Drug-Protein Binding with Machine Learning (AQMA)" aims to combine Quantum Mechanics and Machine Learning approaches for the accurate characterisation of the biochemical systems with the goal of reliable prediction of protein-ligand binding affinity. The research involves performing rigorous tests of machine learning force fields, e.g. TEA Challenge 2023 (10.1039/D4SC06529H and 10.1039/D4SC06530A) as well as developing a proxy model for protein-ligand interactions via molecular dimers QUID with high-fidelity optimisation and reference data of interaction energy among other physicochemical properties (10.26434/chemrxiv-2025-f6615).
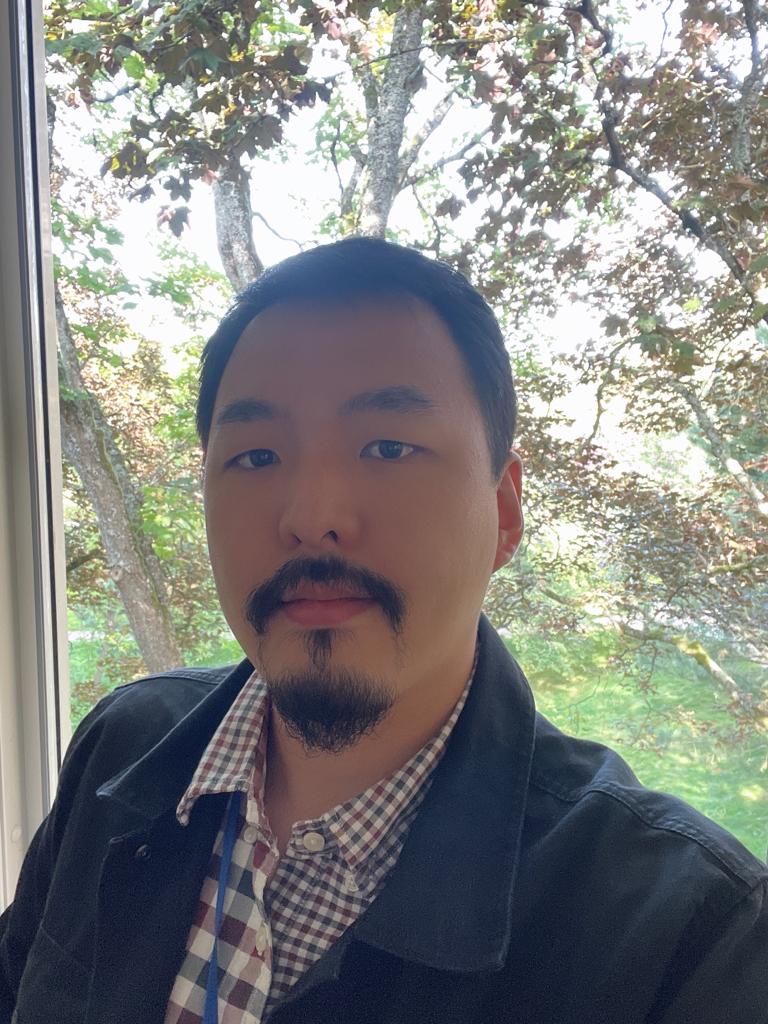
Kyunghoon Han
PhD Student
Current Research: Molecular dynamics (both theoretical and computational), Neural network models for computing thermodynamic potential, Computational simulation of molecular systems
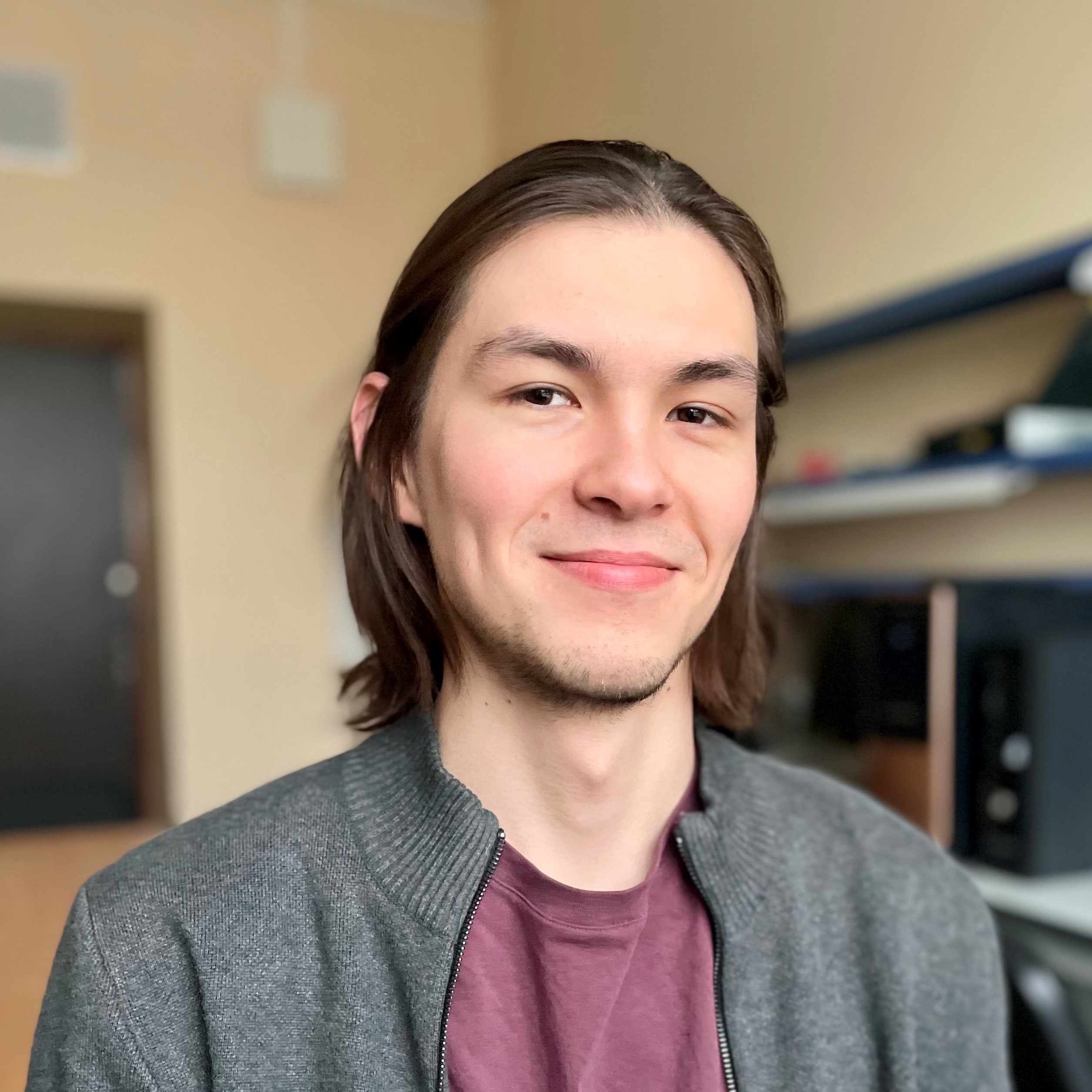
Adil Kabylda
PhD Student
I received my B.Sc. and M.Sc. in Chemistry from Moscow State University in 2021. As an undergraduate, I pursued research in organic synthesis, and during my Master's, I studied fundamental chemical processes in living organisms using state-of-the-art quantum chemistry methods. Currently, my research focuses on extending the applicability of Machine Learning Force Fields to larger molecules, with particular emphasis on accurately describing long-range interactions. My ultimate goal is to enable atomistic-level modeling and understanding of increasingly complex (bio)molecular systems. I grew up in Pavlodar, Kazakhstan, where I discovered my passion for natural sciences through high school chemistry Olympiads. Besides research, my interests include counter-strike, chess, and russian rap music.
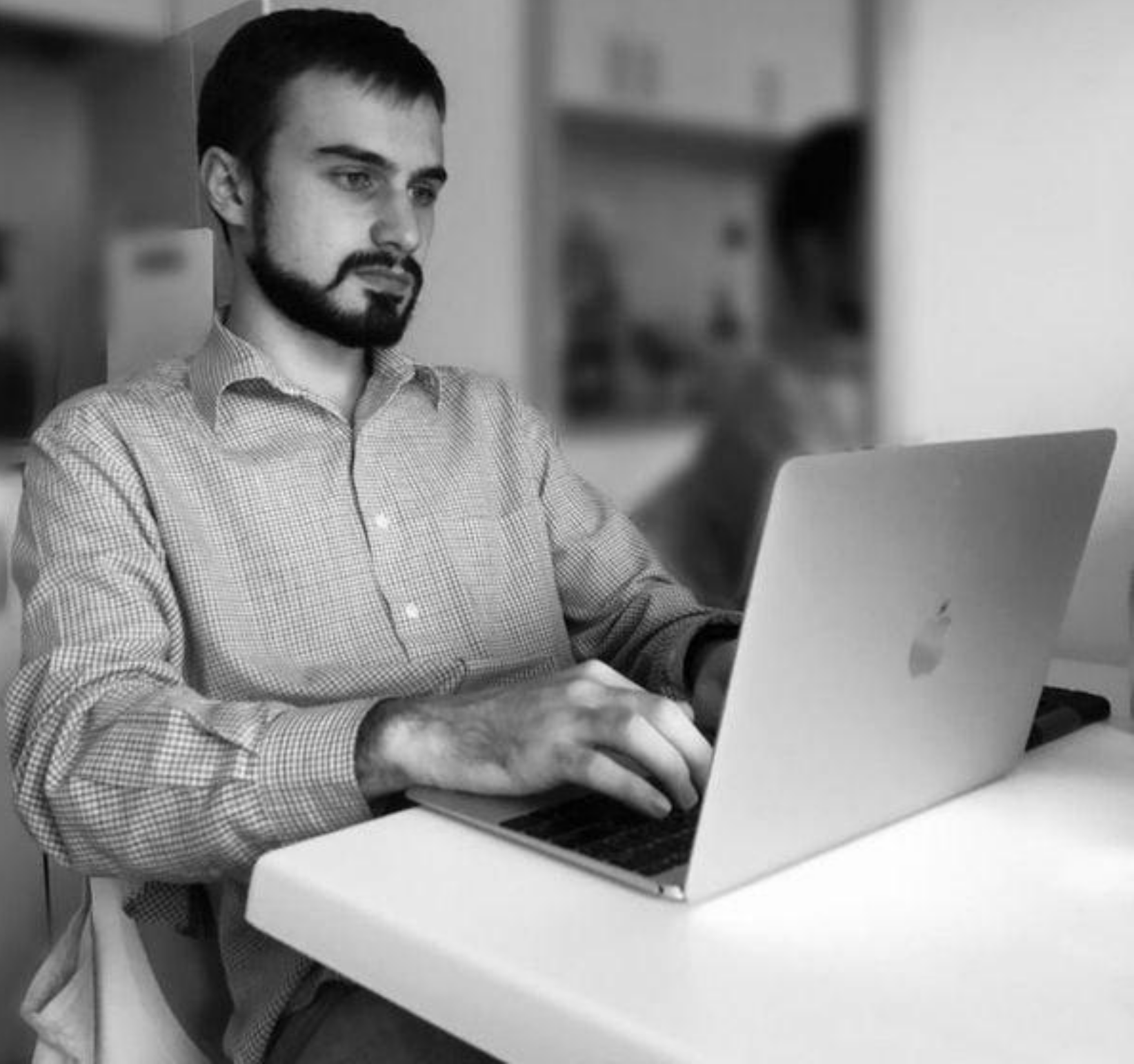
Anton Charkin-Gorbulin
PhD Student
Current Interests: Development of machine learning force fields to study layered halide perovskites in collaboration with the University of Mons.
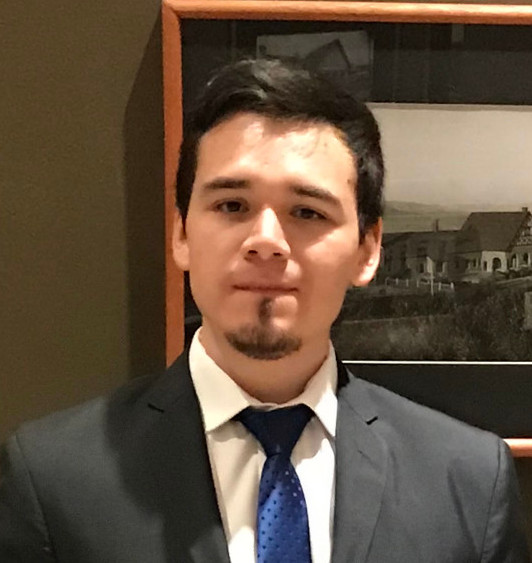
Raul Ian Sosa
PhD Student
I'm a physicist, A.I. developer, and entrepreneur from Argentina. I earned my integrated Licentiate degree (equivalent to a BSc+MSc in Physics) from the University of Buenos Aires and the Balseiro Institute, graduating with the highest honors as the valedictorian in 2019. I then completed a MSc degree in Statistical Physics and Chaos in 2021. Since May 2022, I have been pursuing a PhD under the FNR project Quantum Continuum Coupling (QuaC), working with Prof. Alexandre Tkatchenko to develop multiscale modeling techniques that incorporate quantum effects into engineering-scale systems, with a focus on capturing many-body van der Waals interactions. Alongside academia, I co-founded NeuralWave Technologies, a company focused on A.I.-driven voice synthesis. I also serve on the organizing committee of the European Association for Data Science (EuADS). I enjoy any topic related to deep learning, parallel computing, bioinformatics, and astrophotography!.
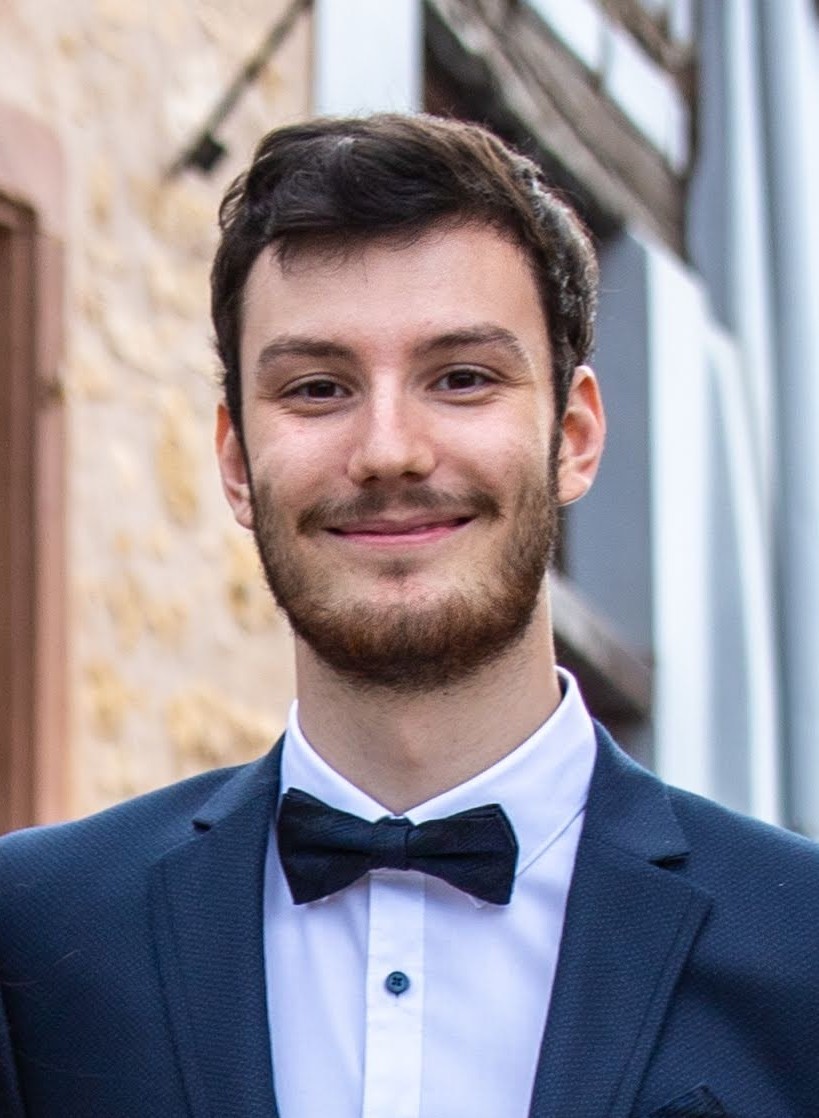
Nils Davoine
PhD Student
Research topics: Predict with machine learning algorithm forces & energies for complex biomolecular systems learning from DFT calculations. Positive results would be matching a relatively good accuracy and a competitive computational time regarding the calculation methods.
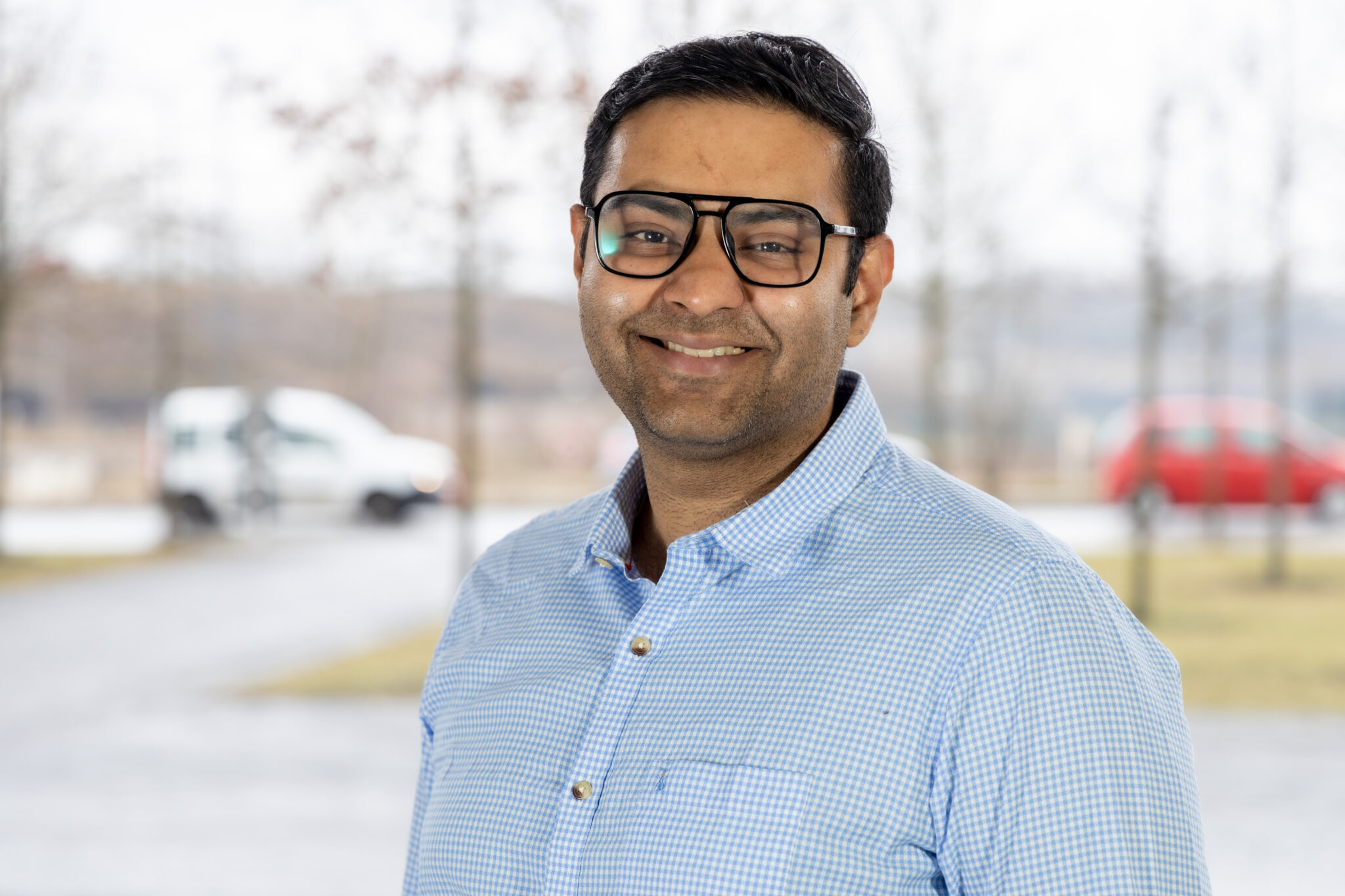
Dhruv Sharma
PhD Student
Research Interests: Non-adiabatic Dynamics. Mixed Quantum-Classical Methods, PT Symmetry, Non-Hermitian Quantum Mechanics, Path-Integral Methods, General Relativity, Gravitational Waves, Black hole scattering
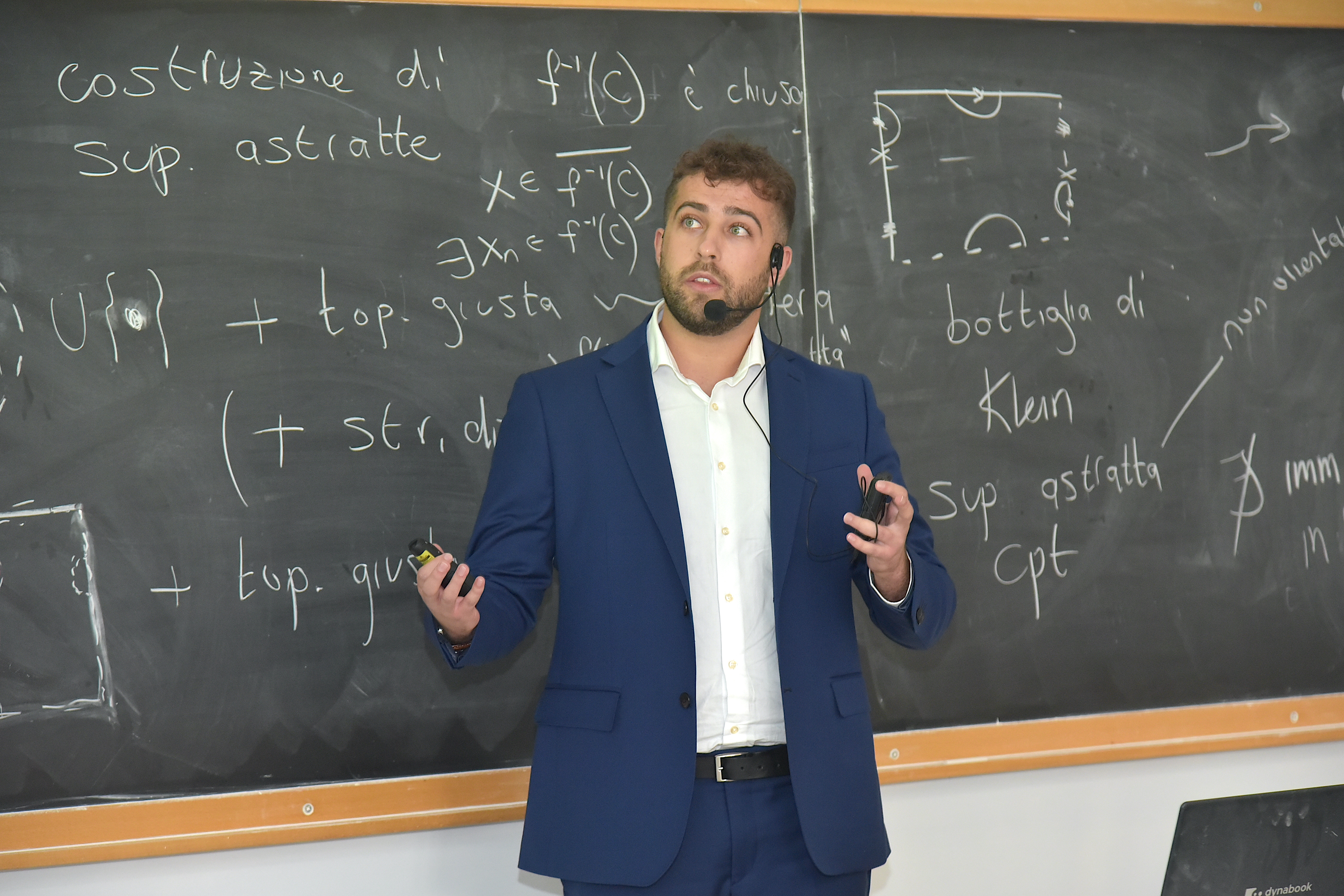
Mathias Hilfiker
PhD Student
Research Topics: Machine-learned quantum Force Fields for molecule-protein interactions.
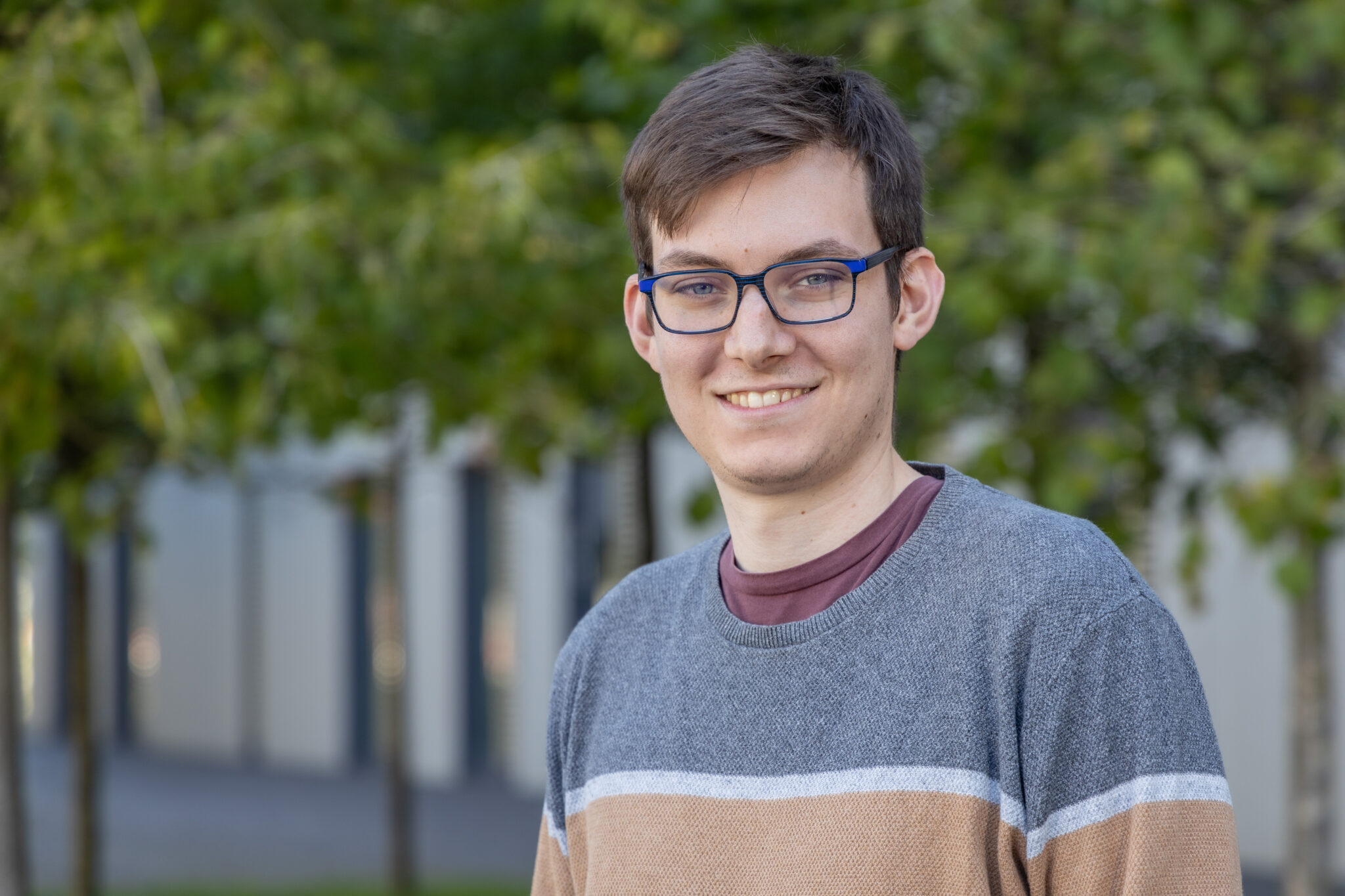
Sergio Suárez Dou
PhD Student
My research focuses on developing and applying machine learning force fields (MLFF) to study complex molecular systems with quantum-level accuracy and enhanced computational efficiency. By combining molecular dynamics simulations, statistical physics, and data-driven methodologies, I aim to understand the conformational space of biomolecules, driving advancements in drug discovery and biomolecular design. I would like to bridge theoretical chemistry with real-world challenges, providing predictive insights into the molecular interactions that reveal biological and chemical processes.
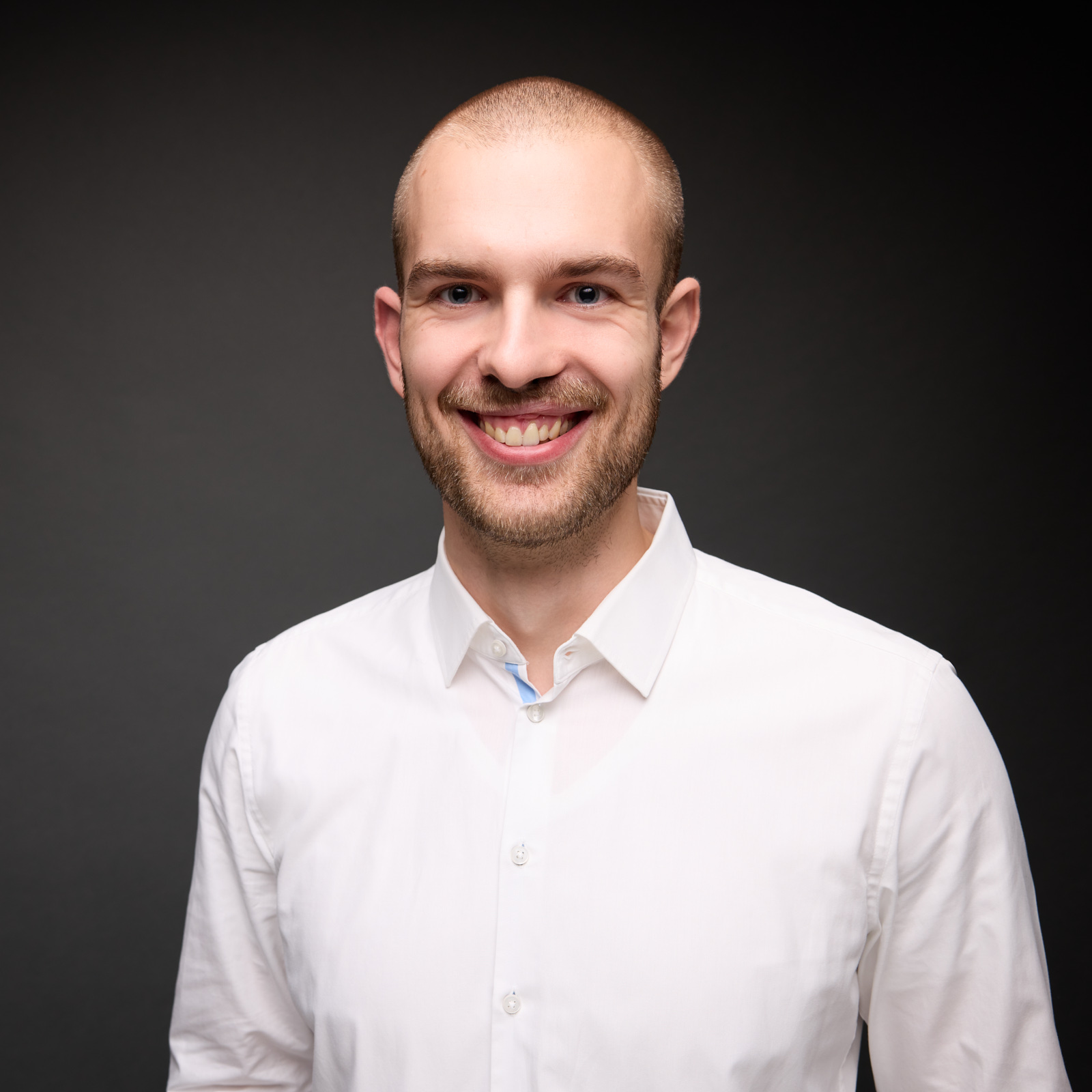
Tobias Henkes
PhD Student
As a chemist, my interests lie in the application, analysis, and automation of machine learning models within the field of molecular simulation. After graduating with a B.Sc. in chemistry from Bielefeld University, Germany, I joined the R&D lab of tesa SE in Hamburg. Afterward, I started my master's studies in chemistry at Saarland University, Germany, during which I joined Prof. Dr. Ernzerhof at the Université de Montréal, Canada. Here, I worked on the application of neural networks to density functional theory and novel electronic structure methods. Since my master's thesis, also completed in the TCP group, I have focused my research on machine learning force fields (MLFFs). In addition to the analysis of so-called foundational MLFFs, a large part of my work is concerned with the automatic and efficient construction of MLFF models and datasets using active learning.